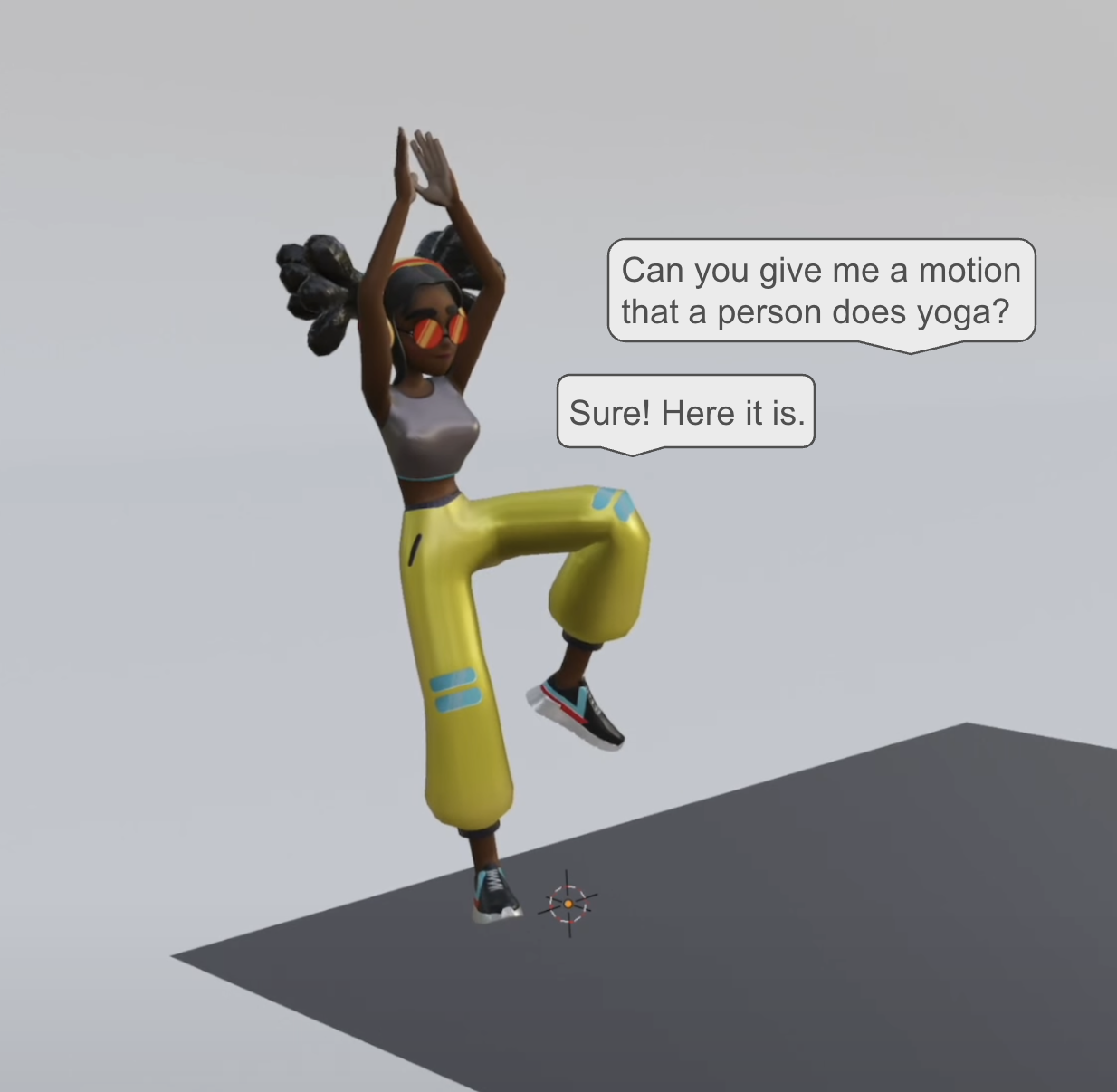
Multimodal model for motion generateion
Train and delploy billion-parameter level language-motion multimodal models that generate realistic 3D human motions from text.
I'm a Research Scientist at Intel Labs. My primary research areas are deep learning and humanoid robots. I lead efforts in developing atheletic and cognitive intelligence for both real robots and virtual characters. To achieve this, I train large multimodal models that understand the world through language, vision and physics. These models are capable of performing complex tasks in simulated environments, with the ultimate goal of transferring these learned skills to real-world robots.
Prior to this, I received my PhD from Penn State, where I was advised by Prof. Bo Cheng. My dissertation work was at the intersection of robot learning and agile locomotion. During my graduate studies, I was fortunate to intern at Intel Labs and MathWorks.
I’ve had the privilege of collaborating with some of the most talented researchers and engineers, including Vladlen Koltun, German Ros, and Alan Fern, and I am always grateful for that experience.
Recent projects in LLM, multimodal model and robotics.
Train and delploy billion-parameter level language-motion multimodal models that generate realistic 3D human motions from text.
Learn control polices for physically realistic bipedal locomotion for humanoid robot, and transfer them to real robots.
Develop an imitation learning framework that learns diverse human motions using massively parallel simulation
(* equal contribution)
Keywords: humanoid motion generation, multimodal, imitation
Keywords: policy gradient, vision-guided inverted landing, nonlinear geometric control, quadrotor, physics-based simulation
Keywords: optical flow, inverted landing, flapping wing aerodynamics, aggressive maneuvers
Keywords: policy search, real-time learning, flapping wing robot, dynamically scale